Tools
We tackle the challenges of data-intensive biology, which span data collection, data interpretation, hypothesis generation, and hypothesis testing. These challenges are biological (e.g., sensitively discovering biological patterns), computational (e.g., effectively integrating heterogeneous data), analytical (e.g., effortlessly generating testable hypotheses), statistical (e.g., confidently teasing out hypotheses without drowning in false positives), and even ecological (e.g., elaborately building reusable software tools).
Featured
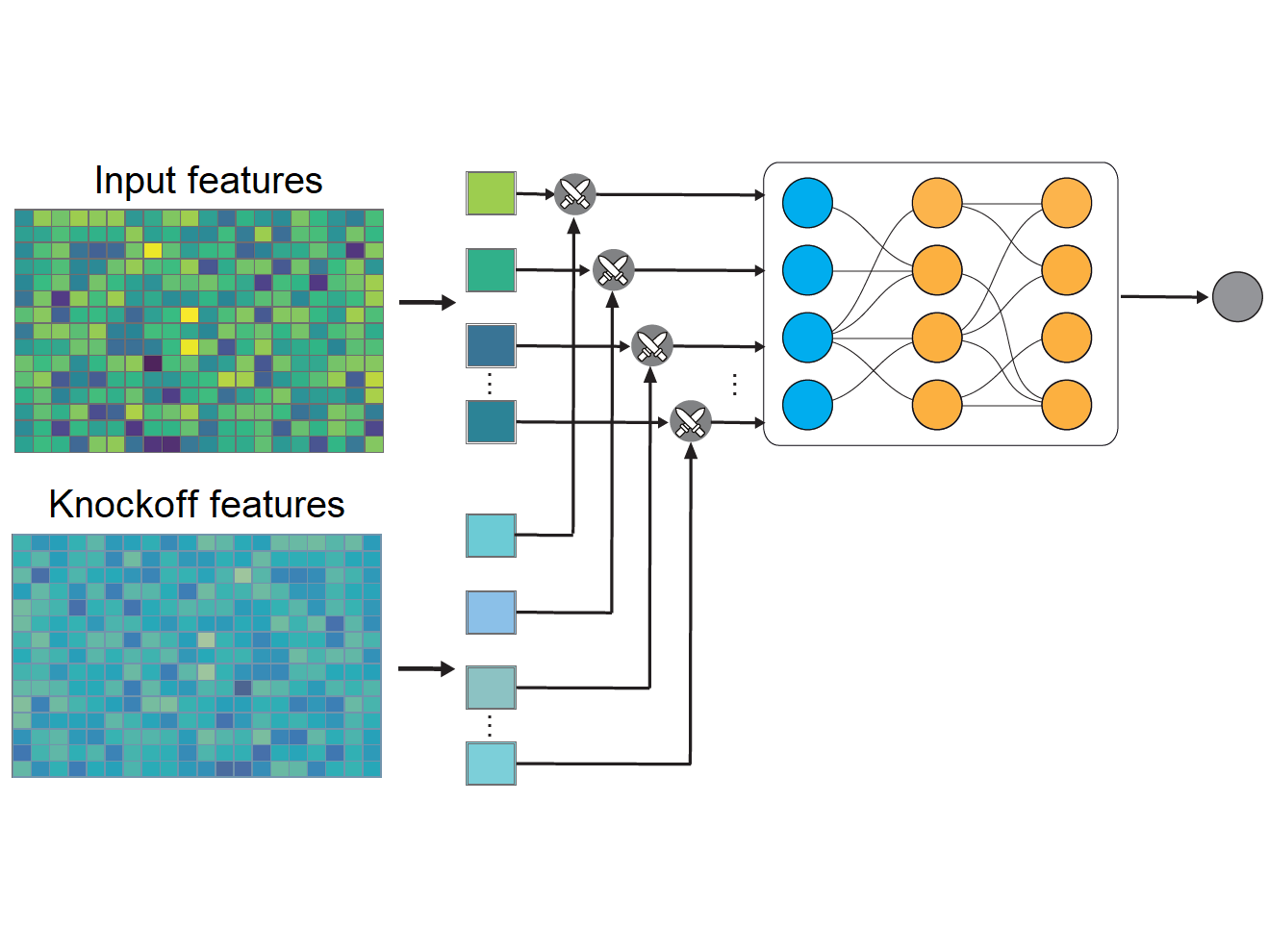
We demonstrated for the first time that the interpretation of deep learning models could achieve statistical guarantees.
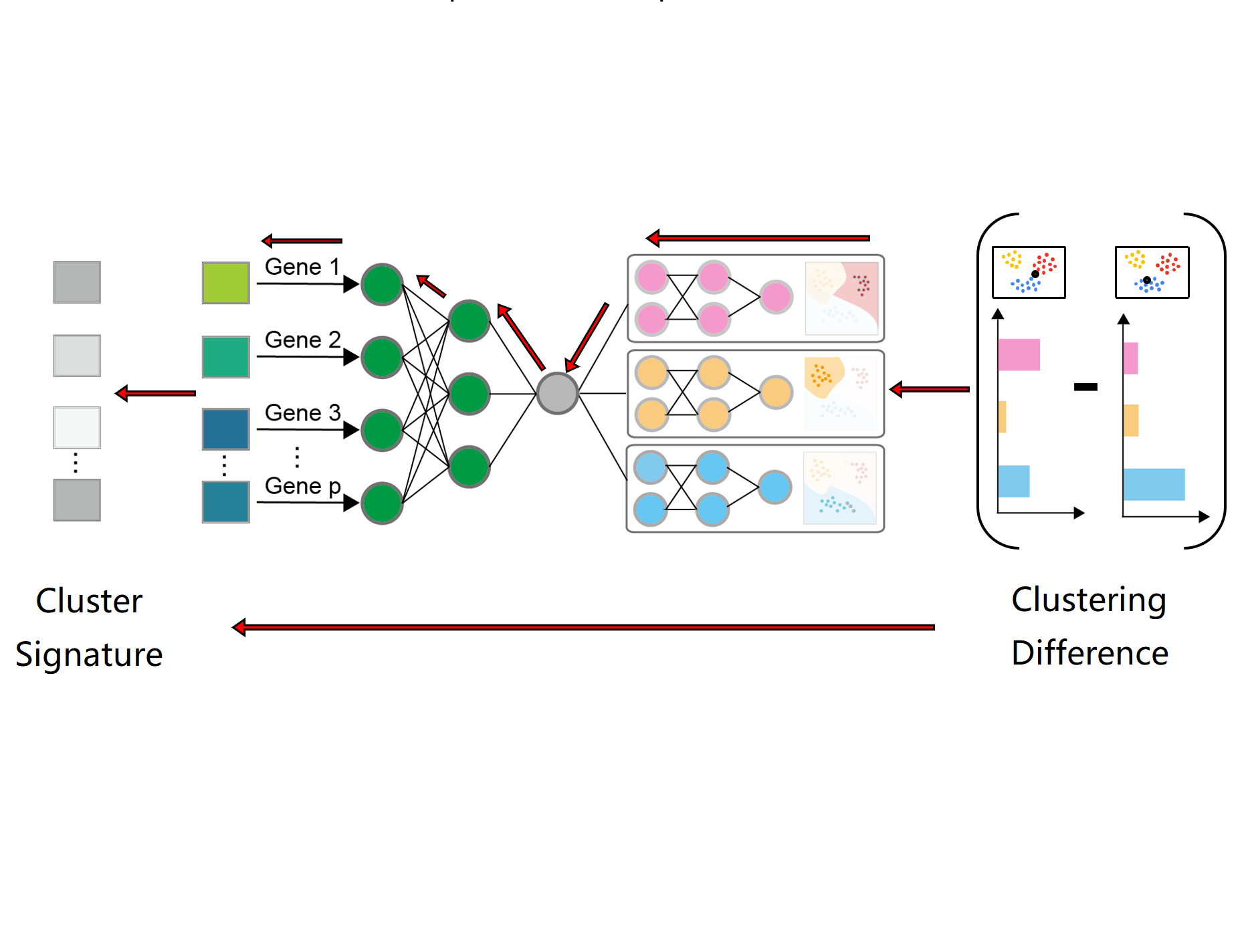
We neuralize the conventional procedure in scRNA-seq analysis and identify gene signatures that are both highly discriminative and less redundant.
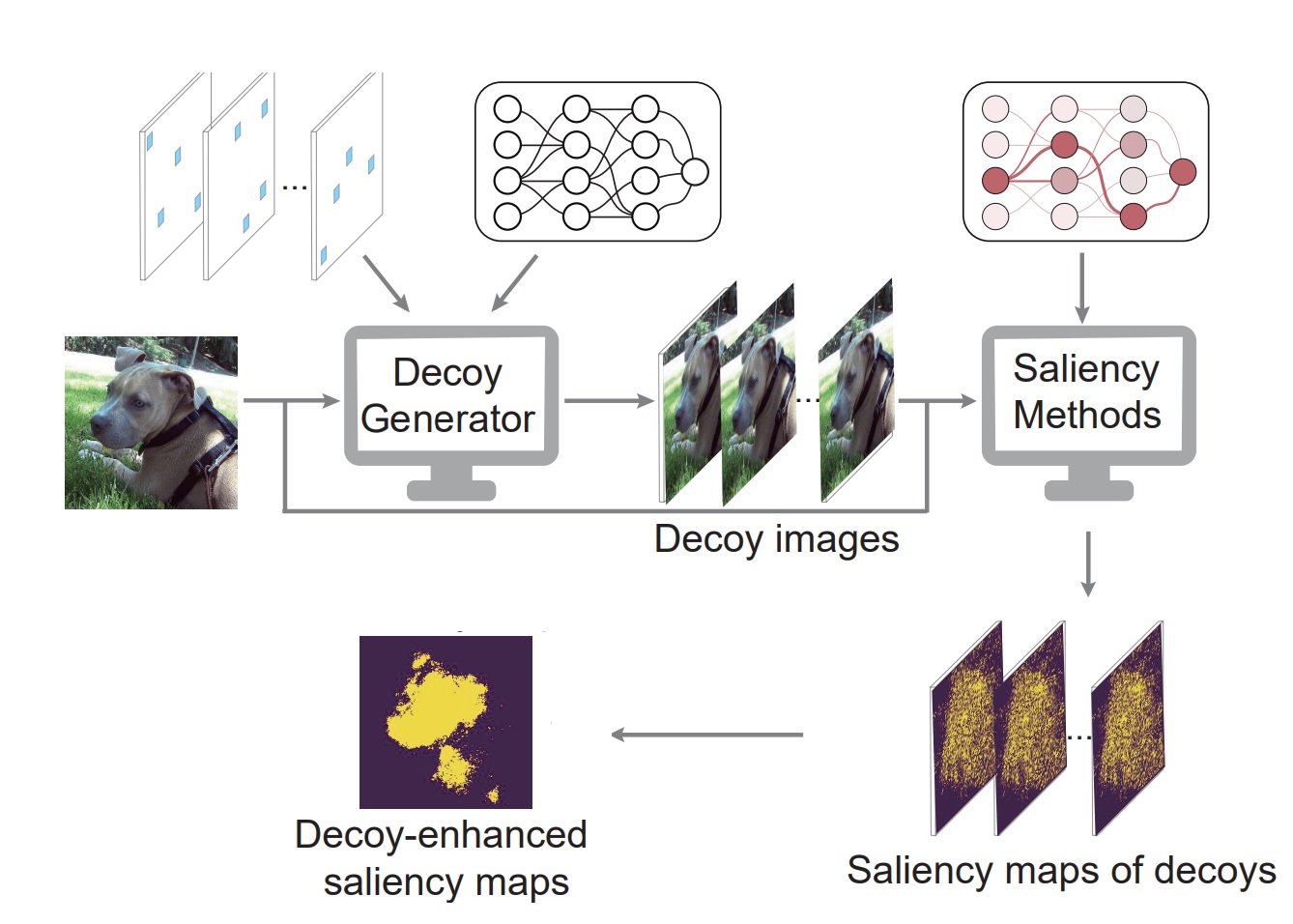
we proposed a more accurate and robust saliency method with theoretical guarantee.
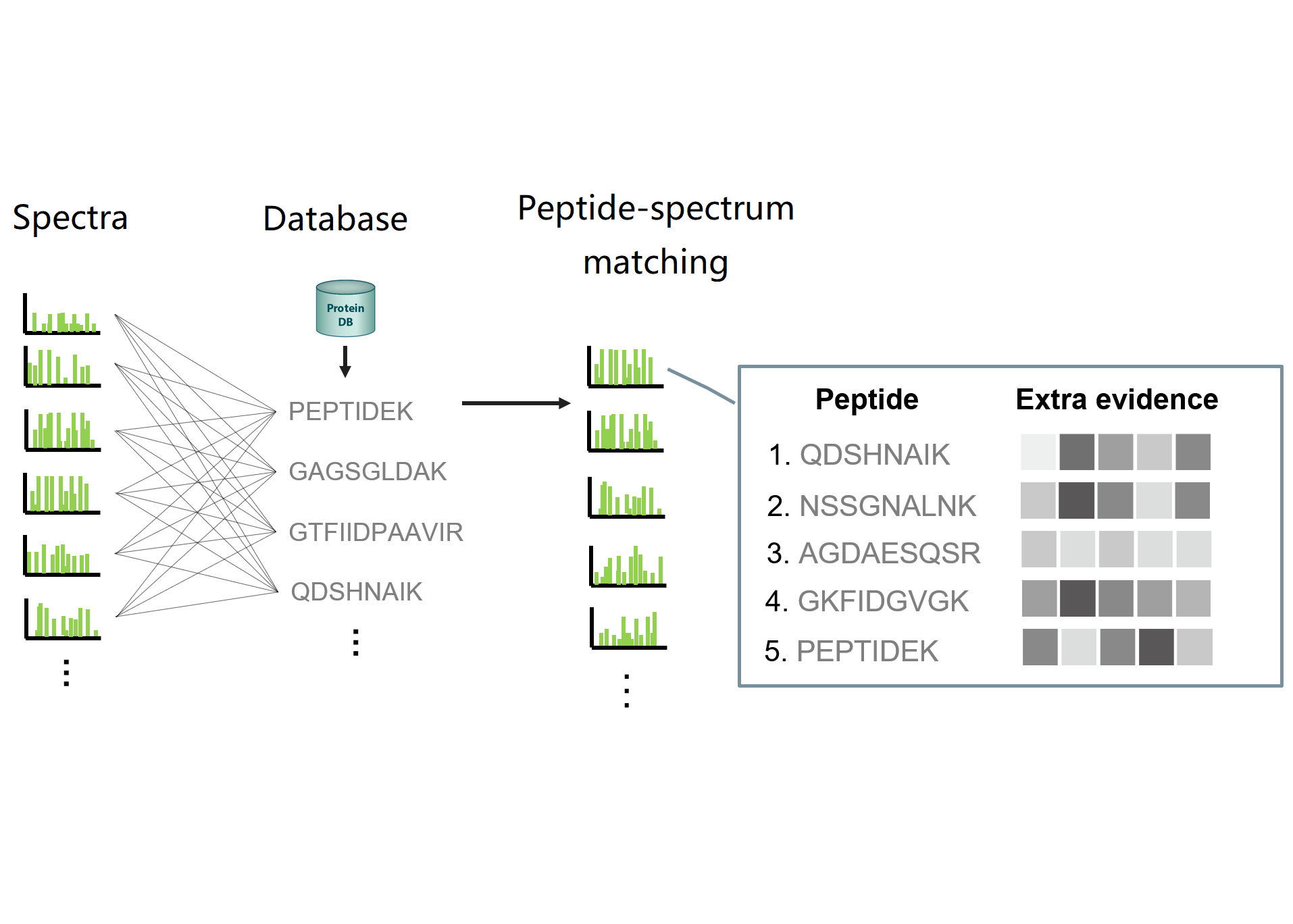
We demonstrated for the first time that protein identification can achieve both sensitivity and reproducibility.
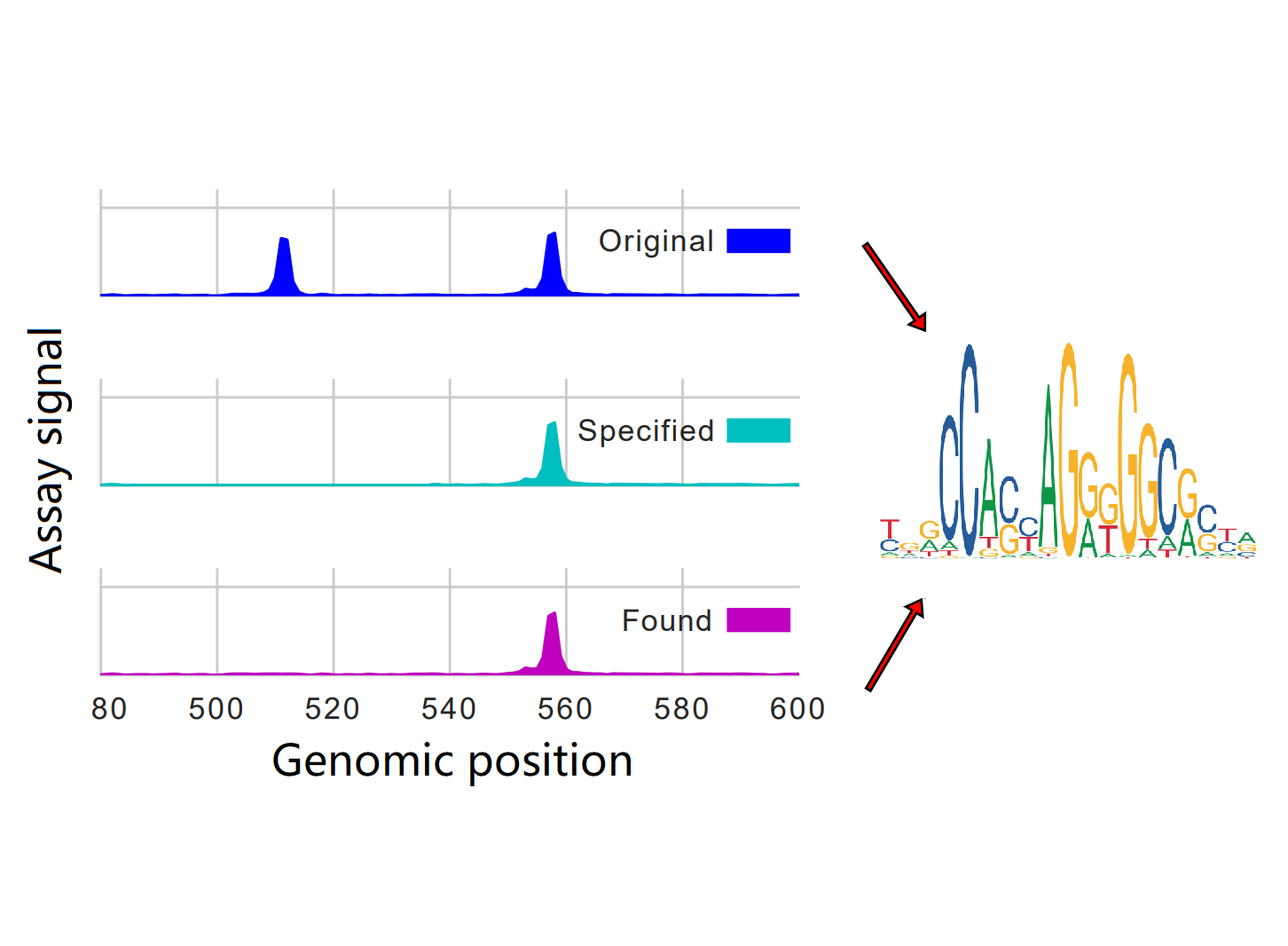
We treated the design of genomic edits as an optimization problem where the goal is to produce the desired output from a specified predictive model.
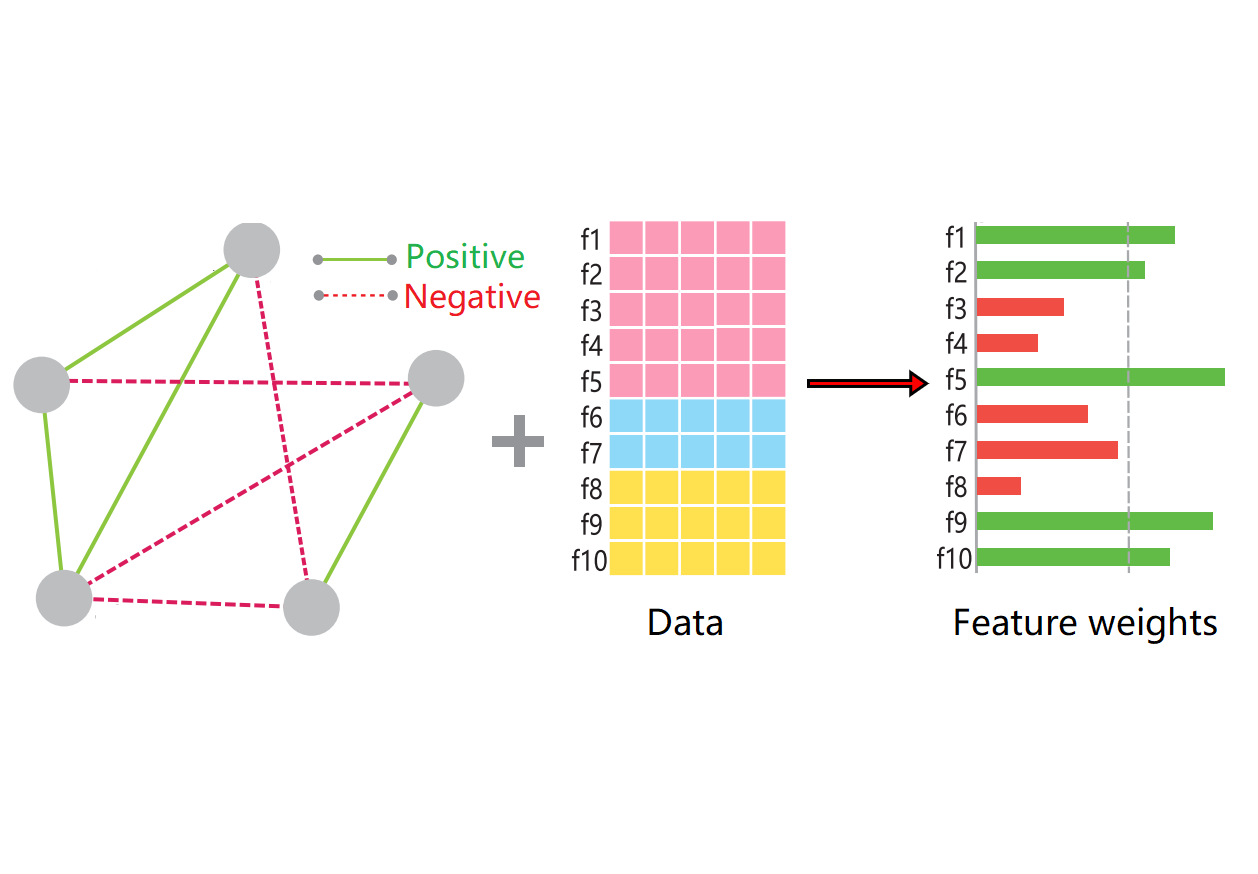
We developed an integrative method that rescales features from heterogeneous data sources.
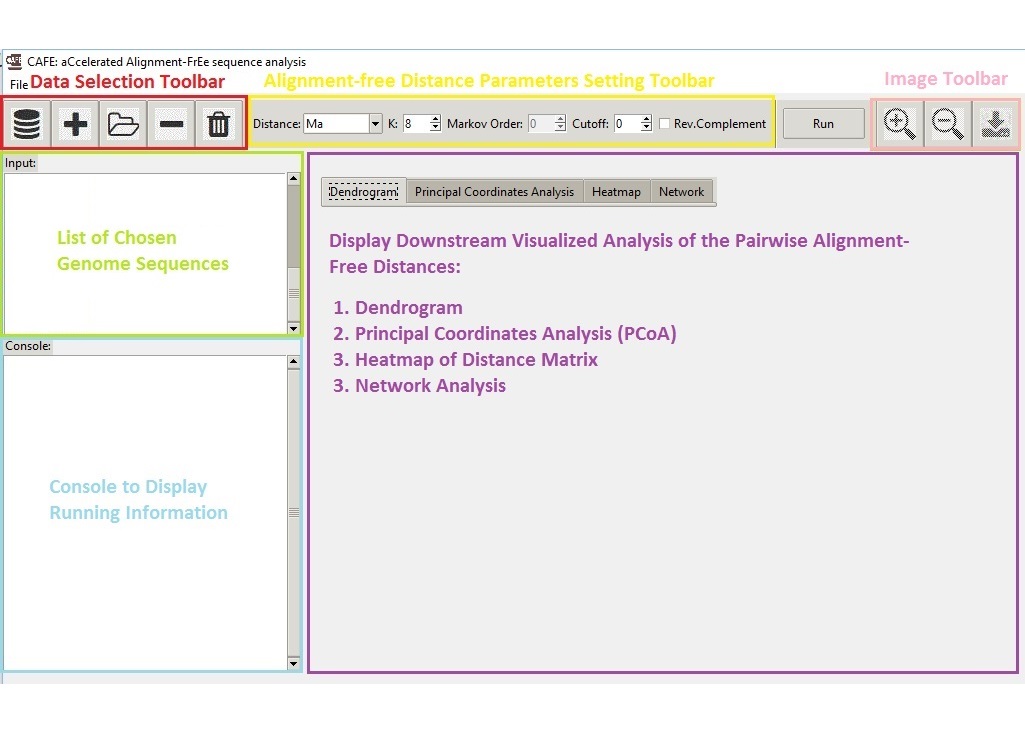
We developed a standalone software for efficient calculation of 28 alignment-free dissimilarity measures.
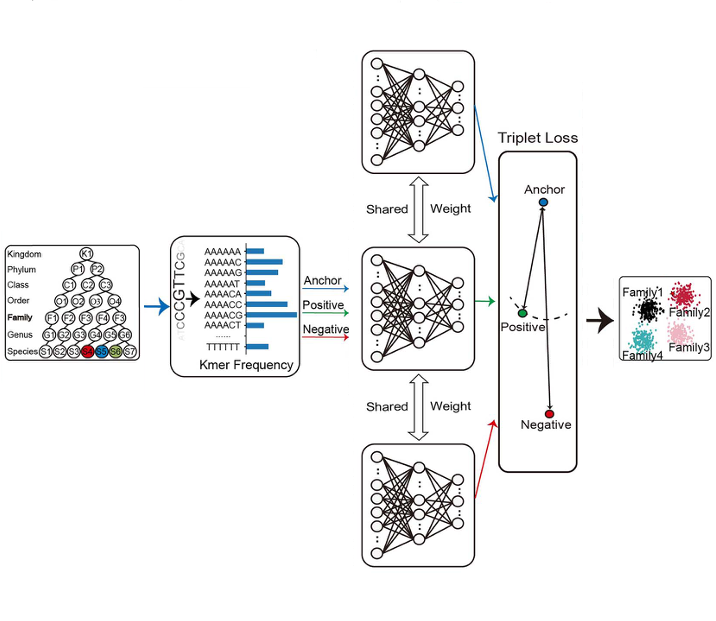
We developed a weakly supervised method for comparative genomics in the absence of label information.